🔖
【Microsoft/python】- MarkItDownを触ってみる
執筆日
2024/12/23
やること
Microsoftが発表したMarkItDownを触ってみる
以下のファイルをMarkdown形式に変換ができるとのこと。
- PDF (.pdf)
- PowerPoint (.pptx)
- Word (.docx)
- Excel (.xlsx)
- Images (EXIF metadata, and OCR)
- Audio (EXIF metadata, and speech transcription)
- HTML (special handling of Wikipedia, etc.)
- Various other text-based formats (csv, json, xml, etc.)
Code
Markdown
以下のコードで実行することで、Markdonw出力をすることができます。
main.py
from markitdown import MarkItDown
markitdown = MarkItDown()
result = markitdown.convert("test.xlsx")
print(result.text_content)
画像の説明
以下のコードを実行することで、画像の説明をLLMで行うことができます。
llm.py
from markitdown import MarkItDown
from openai import AzureOpenAI
endpoint = <"endpoint">
key = <"key">
api_version = <"api_version">
aoai_client = AzureOpenAI(azure_endpoint=endpoint, api_key=key, api_version=api_version)
markitdown = MarkItDown(mlm_client=aoai_client, mlm_model=<"model名">)
result = markitdown.convert(<"ファイルパス">)
print(result.text_content)
前提
- プロジェクトをCloneする
git clone https://github.com/microsoft/markitdown.git
- プロジェクトを開き、以下のコマンドを実行する
pip install markitdown
- tests/test_files配下に以下のファイルを作成する
tests/test_file/main.py
from markitdown import MarkItDown
docx_file = <ファイルパス>
markitdown = MarkItDown()
output = markitdown.convert(docx_file)
print(output.text_content)
tests/test_file/llm.py
from markitdown import MarkItDown
docx_file = <ファイルパス>
markitdown = MarkItDown()
output = markitdown.convert(docx_file)
print(output.text_content)
検証する
/test_files配下のドキュメントで検証を行う。
test_blog.html
おそらく以下のHTMLファイル
結果
<!-- Slide number: 1 -->
# AutoGen: Enabling Next-Gen LLM Applications via Multi-Agent Conversation
Qingyun Wu , Gagan Bansal , Jieyu Zhang, Yiran Wu, Beibin Li, Erkang Zhu, Li Jiang, Xiaoyun Zhang, Shaokun Zhang, Jiale Liu, Ahmed Awadallah, Ryen W. White, Doug Burger, Chi Wang
<!-- Slide number: 2 -->
# 2cdda5c8-e50e-4db4-b5f0-9722a649f455
AutoGen is an open-source framework that allows developers to build LLM applications via multiple agents that can converse with each other to accomplish tasks. AutoGen agents are customizable, conversable, and can operate in various modes that employ combinations of LLMs, human inputs, and tools. Using AutoGen, developers can also flexibly define agent interaction behaviors. Both natural language and 04191ea8-5c73-4215-a1d3-1cfb43aaaf12 can be used to program flexible conversation patterns for different applications. AutoGen serves as a generic framework for building diverse applications of various complexities and LLM capacities. Empirical studies demonstrate the effectiveness of the framework in many example applications, with domains ranging from mathematics, coding, question answering, operations research, online decision-making, entertainment, etc.

<!-- Slide number: 3 -->
# A table to test parsing:
| ColA | ColB | ColC | ColD | ColE | ColF |
| --- | --- | --- | --- | --- | --- |
| 1 | 2 | 3 | 4 | 5 | 6 |
| 7 | 8 | 9 | 1b92870d-e3b5-4e65-8153-919f4ff45592 | 11 | 12 |
| 13 | 14 | 15 | 16 | 17 | 18 |
PS C:\Users\takekawa\Desktop\markitdown\tests\test_files>
PS C:\Users\takekawa\Desktop\markitdown\tests\test_files>
PS C:\Users\takekawa\Desktop\markitdown\tests\test_files> py .\main.py
C:\Users\takekawa\AppData\Local\Programs\Python\Python311\Lib\site-packages\pydub\utils.py:170: RuntimeWarning: Couldn't find ffmpeg or avconv - defaulting to ffmpeg, but may not work
warn("Couldn't find ffmpeg or avconv - defaulting to ffmpeg, but may not work", RuntimeWarning)
[Skip to main content](#__docusaurus_skipToContent_fallback)What's new in AutoGen? Read [this blog](/autogen/blog/2024/03/03/AutoGen-Update) for an overview of updates[**AutoGen**](/autogen/)[Docs](/autogen/docs/Getting-Started)[API](/autogen/docs/reference/agentchat/conversable_agent)[Blog](/autogen/blog)[FAQ](/autogen/docs/FAQ)[Examples](/autogen/docs/Examples)[Notebooks](/autogen/docs/notebooks)[Gallery](/autogen/docs/Gallery)Other Languages
* [Dotnet](https://microsoft.github.io/autogen-for-net/)
[GitHub](https://github.com/microsoft/autogen)`ctrl``K`Recent posts
* [What's New in AutoGen?](/autogen/blog/2024/03/03/AutoGen-Update)
* [StateFlow - Build LLM Workflows with Customized State-Oriented Transition Function in GroupChat](/autogen/blog/2024/02/29/StateFlow)
* [FSM Group Chat -- User-specified agent transitions](/autogen/blog/2024/02/11/FSM-GroupChat)
* [Anny: Assisting AutoGen Devs Via AutoGen](/autogen/blog/2024/02/02/AutoAnny)
* [AutoGen with Custom Models: Empowering Users to Use Their Own Inference Mechanism](/autogen/blog/2024/01/26/Custom-Models)
* [AutoGenBench -- A Tool for Measuring and Evaluating AutoGen Agents](/autogen/blog/2024/01/25/AutoGenBench)
* [Code execution is now by default inside docker container](/autogen/blog/2024/01/23/Code-execution-in-docker)
* [All About Agent Descriptions](/autogen/blog/2023/12/29/AgentDescriptions)
* [AgentOptimizer - An Agentic Way to Train Your LLM Agent](/autogen/blog/2023/12/23/AgentOptimizer)
* [AutoGen Studio: Interactively Explore Multi-Agent Workflows](/autogen/blog/2023/12/01/AutoGenStudio)
* [Agent AutoBuild - Automatically Building Multi-agent Systems](/autogen/blog/2023/11/26/Agent-AutoBuild)
* [How to Assess Utility of LLM-powered Applications?](/autogen/blog/2023/11/20/AgentEval)
* [AutoGen Meets GPTs](/autogen/blog/2023/11/13/OAI-assistants)
* [EcoAssistant - Using LLM Assistants More Accurately and Affordably](/autogen/blog/2023/11/09/EcoAssistant)
* [Multimodal with GPT-4V and LLaVA](/autogen/blog/2023/11/06/LMM-Agent)
* [AutoGen's Teachable Agents](/autogen/blog/2023/10/26/TeachableAgent)
* [Retrieval-Augmented Generation (RAG) Applications with AutoGen](/autogen/blog/2023/10/18/RetrieveChat)
* [Use AutoGen for Local LLMs](/autogen/blog/2023/07/14/Local-LLMs)
* [MathChat - An Conversational Framework to Solve Math Problems](/autogen/blog/2023/06/28/MathChat)
* [Achieve More, Pay Less - Use GPT-4 Smartly](/autogen/blog/2023/05/18/GPT-adaptive-humaneval)
* [Does Model and Inference Parameter Matter in LLM Applications? - A Case Study for MATH](/autogen/blog/2023/04/21/LLM-tuning-math)
# Does Model and Inference Parameter Matter in LLM Applications? - A Case Study for MATH
April 21, 2023 · 6 min read[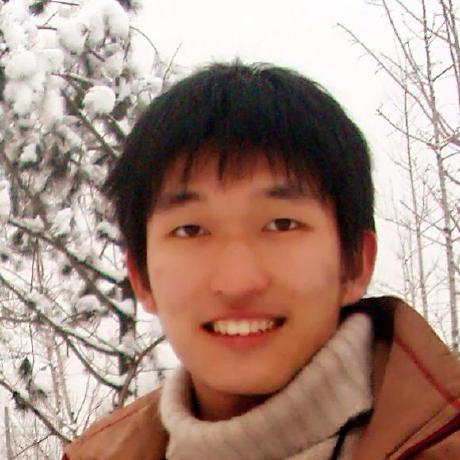](https://www.linkedin.com/in/chi-wang-49b15b16/)[Chi Wang](https://www.linkedin.com/in/chi-wang-49b15b16/)Principal Researcher at Microsoft Research

**TL;DR:**
* **Just by tuning the inference parameters like model, number of responses, temperature etc. without changing any model weights or prompt, the baseline accuracy of untuned gpt-4 can be improved by 20% in high school math competition problems.**
* **For easy problems, the tuned gpt-3.5-turbo model vastly outperformed untuned gpt-4 in accuracy (e.g., 90% vs. 70%) and cost efficiency. For hard problems, the tuned gpt-4 is much more accurate (e.g., 35% vs. 20%) and less expensive than untuned gpt-4.**
* **AutoGen can help with model selection, parameter tuning, and cost-saving in LLM applications.**
Large language models (LLMs) are powerful tools that can generate natural language texts for various applications, such as chatbots, summarization, translation, and more. GPT-4 is currently the state of the art LLM in the world. Is model selection irrelevant? What about inference parameters?
In this blog post, we will explore how model and inference parameter matter in LLM applications, using a case study for [MATH](https://datasets-benchmarks-proceedings.neurips.cc/paper/2021/hash/be83ab3ecd0db773eb2dc1b0a17836a1-Abstract-round2.html), a benchmark for evaluating LLMs on advanced mathematical problem solving. MATH consists of 12K math competition problems from AMC-10, AMC-12 and AIME. Each problem is accompanied by a step-by-step solution.
We will use AutoGen to automatically find the best model and inference parameter for LLMs on a given task and dataset given an inference budget, using a novel low-cost search & pruning strategy. AutoGen currently supports all the LLMs from OpenAI, such as GPT-3.5 and GPT-4.
We will use AutoGen to perform model selection and inference parameter tuning. Then we compare the performance and inference cost on solving algebra problems with the untuned gpt-4. We will also analyze how different difficulty levels affect the results.
## Experiment Setup[](#experiment-setup "Direct link to Experiment Setup")
We use AutoGen to select between the following models with a target inference budget $0.02 per instance:
* gpt-3.5-turbo, a relatively cheap model that powers the popular ChatGPT app
* gpt-4, the state of the art LLM that costs more than 10 times of gpt-3.5-turbo
We adapt the models using 20 examples in the train set, using the problem statement as the input and generating the solution as the output. We use the following inference parameters:
* temperature: The parameter that controls the randomness of the output text. A higher temperature means more diversity but less coherence. We search for the optimal temperature in the range of [0, 1].
* top\_p: The parameter that controls the probability mass of the output tokens. Only tokens with a cumulative probability less than or equal to top-p are considered. A lower top-p means more diversity but less coherence. We search for the optimal top-p in the range of [0, 1].
* max\_tokens: The maximum number of tokens that can be generated for each output. We search for the optimal max length in the range of [50, 1000].
* n: The number of responses to generate. We search for the optimal n in the range of [1, 100].
* prompt: We use the template: "{problem} Solve the problem carefully. Simplify your answer as much as possible. Put the final answer in \boxed{{}}." where {problem} will be replaced by the math problem instance.
In this experiment, when n > 1, we find the answer with highest votes among all the responses and then select it as the final answer to compare with the ground truth. For example, if n = 5 and 3 of the responses contain a final answer 301 while 2 of the responses contain a final answer 159, we choose 301 as the final answer. This can help with resolving potential errors due to randomness. We use the average accuracy and average inference cost as the metric to evaluate the performance over a dataset. The inference cost of a particular instance is measured by the price per 1K tokens and the number of tokens consumed.
## Experiment Results[](#experiment-results "Direct link to Experiment Results")
The first figure in this blog post shows the average accuracy and average inference cost of each configuration on the level 2 Algebra test set.
Surprisingly, the tuned gpt-3.5-turbo model is selected as a better model and it vastly outperforms untuned gpt-4 in accuracy (92% vs. 70%) with equal or 2.5 times higher inference budget.
The same observation can be obtained on the level 3 Algebra test set.

However, the selected model changes on level 4 Algebra.

This time gpt-4 is selected as the best model. The tuned gpt-4 achieves much higher accuracy (56% vs. 44%) and lower cost than the untuned gpt-4.
On level 5 the result is similar.

We can see that AutoGen has found different optimal model and inference parameters for each subset of a particular level, which shows that these parameters matter in cost-sensitive LLM applications and need to be carefully tuned or adapted.
An example notebook to run these experiments can be found at: <https://github.com/microsoft/FLAML/blob/v1.2.1/notebook/autogen_chatgpt.ipynb>. The experiments were run when AutoGen was a subpackage in FLAML.
## Analysis and Discussion[](#analysis-and-discussion "Direct link to Analysis aand Discussion")
While gpt-3.5-turbo demonstrates competitive accuracy with voted answers in relatively easy algebra problems under the same inference budget, gpt-4 is a better choice for the most difficult problems. In general, through parameter tuning and model selection, we can identify the opportunity to save the expensive model for more challenging tasks, and improve the overall effectiveness of a budget-constrained system.
There are many other alternative ways of solving math problems, which we have not covered in this blog post. When there are choices beyond the inference parameters, they can be generally tuned via [`flaml.tune`](https://microsoft.github.io/FLAML/docs/Use-Cases/Tune-User-Defined-Function).
The need for model selection, parameter tuning and cost saving is not specific to the math problems. The [Auto-GPT](https://github.com/Significant-Gravitas/Auto-GPT) project is an example * [Research paper about the tuning technique](https://arxiv.org/abs/2303.04673)
* [Documentation about inference tuning](/autogen/docs/Use-Cases/enhanced_inference)
*Do you have any experience to share about LLM applications? Do you like to see more support or research of LLM optimization or automation? Please join our [Discord](https://discord.gg/pAbnFJrkgZ) server for discussion.*
**Tags:**
* [Research paper about the tuning technique](https://arxiv.org/abs/2303.04673)
* [Documentation about inference tuning](/autogen/docs/Use-Cases/enhanced_inference)
*Do you have any experience to share about LLM applications? Do you like to see more support or research of LLM optimization or automation? Please join our [Discord](https://discord.gg/pAbnFJrkgZ) server for discussion.*
**Tags:**
* [LLM](/autogen/blog/tags/llm)
* [GPT](/autogen/blog/tags/gpt)
* [Documentation about inference tuning](/autogen/docs/Use-Cases/enhanced_inference)
*Do you have any experience to share about LLM applications? Do you like to see more support or research of LLM optimization or automation? Please join our [Discord](https://discord.gg/pAbnFJrkgZ) server for discussion.*
**Tags:**
*Do you have any experience to share about LLM applications? Do you like to see more support or research of LLM optimization or automation? Please join our [Discord](https://discord.gg/pAbnFJrkgZ) server for discussion.*
**Tags:**
nFJrkgZ) server for discussion.*
**Tags:**
* [LLM](/autogen/blog/tags/llm)
* [GPT](/autogen/blog/tags/gpt)
* [LLM](/autogen/blog/tags/llm)
* [GPT](/autogen/blog/tags/gpt)
* [research](/autogen/blog/tags/research)
* [GPT](/autogen/blog/tags/gpt)
* [research](/autogen/blog/tags/research)
* [research](/autogen/blog/tags/research)
[Newer PostAchieve More, Pay Less - Use GPT-4 Smartly](/autogen/blog/2023/05/18/GPT-adaptive-humaneval)
[Newer PostAchieve More, Pay Less - Use GPT-4 Smartly](/autogen/blog/2023/05/18/GPT-adaptive-humaneval)
* [Experiment Results](#experiment-results)
* [Analysis and Discussion](#analysis-and-discussion)
* [For Further Reading](#for-further-reading)
Community
* [Discord](https://discord.gg/pAbnFJrkgZ)
* [Twitter](https://twitter.com/pyautogen)
Copyright © 2024 AutoGen Authors | [Privacy and Cookies](https://go.microsoft.com/fwlink/?LinkId=521839)
test.docs
結果
AutoGen: Enabling Next-Gen LLM Applications via Multi-Agent Conversation
Qingyun Wu , Gagan Bansal , Jieyu Zhang, Yiran Wu, Beibin Li, Erkang Zhu, Li Jiang, Xiaoyun Zhang, Shaokun Zhang, Jiale Liu, Ahmed Awadallah, Ryen W. White, Doug Burger, Chi Wang
# Abstract
AutoGen is an open-source framework that allows developers to build LLM applications via multiple agents that can converse with each other to accomplish tasks. AutoGen agents are customizable, conversable, and can operate in various modes that employ combinations of LLMs, human inputs, and tools. Using AutoGen, developers can also flexibly define agent interaction behaviors. Both natural language and computer code can be used to program flexible conversation patterns for different applications. AutoGen serves as a generic framework for building diverse applications of various complexities and LLM capacities. Empirical studies demonstrate the effectiveness of the framework in many example applications, with domains ranging from mathematics, coding, question answering, operations research, online decision-making, entertainment, etc.
# Introduction
Large language models (LLMs) are becoming a crucial building block in developing powerful agents that utilize LLMs for reasoning, tool usage, and adapting to new observations (Yao et al., 2022; Xi et al., 2023; Wang et al., 2023b) in many real-world tasks. Given the expanding tasks that could benefit from LLMs and the growing task complexity, an intuitive approach to scale up the power of agents is to use multiple agents that cooperate. Prior work suggests that multiple agents can help encourage divergent thinking (Liang et al., 2023), improve factuality and reasoning (Du et al., 2023), and provide validation (Wu et al., 2023).
## d666f1f7-46cb-42bd-9a39-9a39cf2a509f
In light of the intuition and early evidence of promise, it is intriguing to ask the following question: how can we facilitate the development of LLM applications that could span a broad spectrum of domains and complexities based on the multi-agent approach? Our insight is to use multi-agent conversations to achieve it. There are at least three reasons confirming its general feasibility and utility thanks to recent advances in LLMs: First, because chat optimized LLMs (e.g., GPT-4) show the ability to incorporate feedback, LLM agents can cooperate through conversations with each other or human(s), e.g., a dialog where agents provide and seek reasoning, observations, critiques, and validation. Second, because a single LLM can exhibit a broad range of capabilities (especially when configured with the correct prompt and inference settings), conversations between differently configured agents can help combine these broad LLM capabilities in a modular and complementary manner. Third, LLMs have demonstrated ability to solve complex tasks when the tasks are broken into simpler subtasks. Here is a random UUID in the middle of the paragraph! 314b0a30-5b04-470b-b9f7-eed2c2bec74a Multi-agent conversations can enable this partitioning and integration in an intuitive manner. How can we leverage the above insights and support different applications with the common requirement of coordinating multiple agents, potentially backed by LLMs, humans, or tools exhibiting different capacities? We desire a multi-agent conversation framework with generic abstraction and effective implementation that has the flexibility to satisfy different application needs. Achieving this requires addressing two critical questions: (1) How can we design individual agents that are capable, reusable, customizable, and effective in multi-agent collaboration? (2) How can we develop a straightforward, unified interface that can accommodate a wide range of agent conversation patterns? In practice, applications of varying complexities may need distinct sets of agents with specific capabilities, and may require different conversation patterns, such as single- or multi-turn dialogs, different human involvement modes, and static vs. dynamic conversation. Moreover, developers may prefer the flexibility to program agent interactions in natural language or code. Failing to adequately address these two questions would limit the framework’s scope of applicability and generality.
Here is a random table for .docx parsing test purposes:
| 1 | 2 | 3 | 4 | 5 | 6 |
| --- | --- | --- | --- | --- | --- |
| 7 | 8 | 9 | 10 | 11 | 12 |
| 13 | 14 | 49e168b7-d2ae-407f-a055-2167576f39a1 | 15 | 16 | 17 |
| 18 | 19 | 20 | 21 | 22 | 23 |
| 24 | 25 | 26 | 27 | 28 | 29 |
test.xlsx
結果
## Sheet1
| Alpha | Beta | Gamma | Delta |
| --- | --- | --- | --- |
| 89 | 82 | 100 | 12 |
| 76 | 89 | 33 | 42 |
| 60 | 84 | 19 | 19 |
| 7 | 69 | 10 | 17 |
| 87 | 89 | 86 | 54 |
| 23 | 4 | 89 | 25 |
| 70 | 84 | 62 | 59 |
| 83 | 37 | 43 | 21 |
| 71 | 15 | 88 | 32 |
| 20 | 62 | 20 | 67 |
| 67 | 18 | 15 | 48 |
| 42 | 5 | 15 | 67 |
| 58 | 6ff4173b-42a5-4784-9b19-f49caff4d93d | 22 | 9 |
| 49 | 93 | 6 | 38 |
| 82 | 28 | 1 | 39 |
| 95 | 55 | 18 | 82 |
| 50 | 46 | 98 | 86 |
| 31 | 46 | 47 | 82 |
| 40 | 65 | 19 | 31 |
| 95 | 65 | 29 | 62 |
| 68 | 57 | 34 | 54 |
| 96 | 66 | 63 | 14 |
| 87 | 93 | 95 | 80 |
## 09060124-b5e7-4717-9d07-3c046eb
| ColA | ColB | ColC | ColD |
| --- | --- | --- | --- |
| 1 | 2 | 3 | 4 |
| 5 | 6 | 7 | 8 |
| 9 | 10 | 11 | 12 |
| 13 | 14 | 15 | affc7dad-52dc-4b98-9b5d-51e65d8a8ad0 |
test.pptx
-
slide1
-
slide2
-
dlide3
結果
<!-- Slide number: 1 -->
# AutoGen: Enabling Next-Gen LLM Applications via Multi-Agent Conversation
Qingyun Wu , Gagan Bansal , Jieyu Zhang, Yiran Wu, Beibin Li, Erkang Zhu, Li Jiang, Xiaoyun Zhang, Shaokun Zhang, Jiale Liu, Ahmed Awadallah, Ryen W. White, Doug Burger, Chi Wang
<!-- Slide number: 2 -->
# 2cdda5c8-e50e-4db4-b5f0-9722a649f455
AutoGen is an open-source framework that allows developers to build LLM applications via multiple agents that can converse with each other to accomplish tasks. AutoGen agents are customizable, conversable, and can operate in various modes that employ combinations of LLMs, human inputs, and tools. Using AutoGen, developers can also flexibly define agent interaction behaviors. Both natural language and 04191ea8-5c73-4215-a1d3-1cfb43aaaf12 can be used to program flexible conversation patterns for different applications. AutoGen serves as a generic framework for building diverse applications of various complexities and LLM capacities. Empirical studies demonstrate the effectiveness of the framework in many example applications, with domains ranging from mathematics, coding, question answering, operations research, online decision-making, entertainment, etc.

<!-- Slide number: 3 -->
# A table to test parsing:
| ColA | ColB | ColC | ColD | ColE | ColF |
| --- | --- | --- | --- | --- | --- |
| 1 | 2 | 3 | 4 | 5 | 6 |
| 7 | 8 | 9 | 1b92870d-e3b5-4e65-8153-919f4ff45592 | 11 | 12 |
| 13 | 14 | 15 | 16 | 17 | 18 |
test.jpg
現時点でサポートしてる拡張子は、".jpg", ".jpeg", ".png" のみ。
画像の説明をLLMで行うこと。
main.py
import os
from dotenv import load_dotenv
from markitdown import MarkItDown
from openai import AzureOpenAI
endpoint = <"endpoint">
key = <"key">
api_version = <"api_version">
aoai_client = AzureOpenAI(azure_endpoint=endpoint, api_key=key, api_version=api_version)
markitdown = MarkItDown(mlm_client=aoai_client, mlm_model=<"model名">)
result = markitdown.convert("./test.jpg")
print(result.text_content)
結果
MLM Prompt:
Write a detailed caption for this image.
# Description:
This image depicts the cover page of a paper titled "AutoGen: Enabling Next-Gen LLM Applications via Multi-Agent Conversation." The paper, authored by researchers from Microsoft Research, Pennsylvania State University, University of Washington, and Xidian University, explores the AutoGen framework. AutoGen is a system that facilitates the development of advanced language model (LLM) applications through multi-agent interactions.
The framework is designed to be customizable and conversable, utilizing agents that can perform tasks by integrating LLMs, human input, and various tools. The image includes a diagram illustrating how AutoGen enables diverse applications using multi-agent conversations. On the left, conversable and customizable agent concepts are shown. In the center, it highlights flexible conversation patterns, and on the right, it gives examples of agent chat applications.
The abstract outlines AutoGen as an open-source framework that simplifies building applications across domains like mathematics, coding, and decision-making by allowing agents to communicate and coordinate task execution. The image also includes the authors' affiliations and contact information.
プロンプトが規定で以下になっているぽい。
MLM Prompt:
Write a detailed caption for this image.
上書きしてみる。
main.py
result = markitdown.convert(
"./test.jpg",
mlm_prompt="Structured output in markdown of the content written in the image",
)
print(result.text_content)
結結果果
MLM Prompt:
Structured output in markdown of the content written in the image
Description:
# AutoGen: Enabling Next-Gen LLM Applications via Multi-Agent Conversation
### Authors
- Qingyun Wu
- Gagan Bansal
- Jieyu Zhang‡
- Yiran Wu†
- Beibin Li*
- Erkang Zhu
- Li Jiang*
- Xiaoyun Zhang*
- Shaokun Zhang†
- Jiale Liu⊥
- Ahmed Awadallah*
- Ryen W. White*
- Doug Burger*
- Chi Wang*¹
**Affiliations:**
- *Microsoft Research
- †Pennsylvania State University
- ‡University of Washington
- ⊥Xidian University
### Figure 1: AutoGen Capabilities
AutoGen enables diverse LLM-based applications using multi-agent conversations:
- **Conservable and Customizable Agents:** Can be based on LLMs, tools, humans, or a combination.
- **Task-Oriented Conversations:** Agents can converse to solve tasks with humans in the loop.
- **Flexible Patterns:** Supports flexible conversation patterns.
### Abstract
AutoGen is an open-source framework for building LLM applications via multiple agents:
- **Features:**
- Customizable and conversable agents.
- Agents can operate in modes that combine LLMs, human input, and tools.
- Flexible agent interaction behaviors can be defined.
- Natural language and code interaction.
- **Framework Utility:**
- Generic framework for building diverse applications.
- Demonstrates effectiveness in domains like mathematics, coding, and more.
¹Corresponding author: Email: auto-gen@outlook.com
²GitHub: [https://github.com/microsoft/autogen](https://github.com/microsoft/autogen)
まとめ
MarkitDownを試してみました。Office系をはじめとする多様なファイル形式をMarkdown形式に変換できる点が非常に便利だなーと感じました。Document IntelligenceのLayoutモデルの代替としても利用できる可能性があると考えています。また、LLM(大規模言語モデル)との連携が可能であるため、今後のLLMの進歩に伴い、さらに有用性が高まりそうだなーと。
Discussion